BeautifulSoup makes it easy to quickly scrape content from web pages. Here are two examples.
Electricity prices from Tocom: https://www.tocom.or.jp/market/kobetu/east_base_elec.html
The page has three blocks (Current, Night, and Day sessions). Each block is under a h3
, with the first table providing the session name and date, and the second table provides the prices. The first table is a bare table consisting of table rows, while the second table has a thead
element.
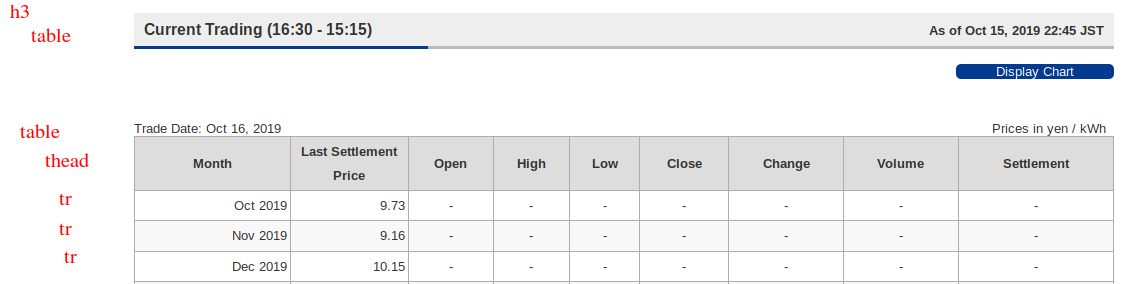
To parse the entire page, we loop through each h3
and use find_next_sibling to get the two tables.
soup = bs4.BeautifulSoup(requests.get(url).content,
features='html.parser')
h3 = soup.find('h3')
while h3 is not None:
name = h3.contents[0].strip()
table0 = h3.find_next_sibling('table')
table1 = table0.find_next_sibling('table')
tables[name] = [parse_rows(table0),
parse_html_table_with_header(table1)]
h3 = h3.find_next_sibling('h3')
To parse a table we can just look for any tr
elements and then pull out all the td
elements. We check if the thing we have supports find_all
by calling hasattr
. This is a quick and dirty way to skip over textual elements between the table rows.
def parse_rows(x):
rows = []
if hasattr(x, 'find_all'):
for row in x.find_all('tr'):
cols = row.find_all('td')
cols =
this_row = ]
if cols:
rows.append(this_row)
return rows
To parse a table with a header, we do the usual for the rows and also search for thead
and all th
elements.
def parse_html_table_with_header(t):
rows = []
for bits in t:
x = parse_rows(bits)
if x != []: rows += x
header = [h.text.strip() for h in \
t.find('thead').find_all('th')]
return (header, rows)
Once we have all the tables it is straightforward to convert it into a Pandas DataFrame. See the full source for how to do this: https://github.com/carlohamalainen/playground/blob/master/python/beautiful_soup_4/tocom_kobetu_prices.py
Sample output:
$ python tocom_kobetu_prices.py
https://www.tocom.or.jp/market/kobetu/east_base_elec.html
Current Trading (16:30 - 15:15)
Trade Date: Oct 16, 2019
Prices in yen / kWh
Month Last Settlement Price Open High Low Close Change Volume Settlement
0 Oct 2019 9.73 - - - - - - -
1 Nov 2019 9.16 - - - - - - -
2 Dec 2019 10.15 - - - - - - -
3 Jan 2020 10.71 - - - - - - -
4 Feb 2020 10.72 - - - - - - -
5 Mar 2020 9.28 - - - - - - -
6 Apr 2020 9.05 - - - - - - -
7 May 2020 9.02 - - - - - - -
8 Jun 2020 9.04 - - - - - - -
9 Jul 2020 10.24 - - - - - - -
10 Aug 2020 10.07 - - - - - - -
11 Sep 2020 9.11 - - - - - - -
12 Oct 2020 8.97 - - - - - - -
13 Nov 2020 8.81 - - - - - - -
14 Dec 2020 9.32 - - - - - - -
The next example is scraping stock prices from Yahoo. I used to use Alphavantage for daily closing prices but their free API doesn’t seem to work at the moment. (Their API says that I have been rate limited, but I was only querying it once a day for a handful of equities).
Luckily for us, the historical pages on Yahoo have a json blob in the middle with all the info that we need, so we can avoid parsing HTML tables. We just grab the content after root.App.main
mg and parse as json:
base = 'https://sg.finance.yahoo.com/quote'
ticker = 'BHP.AX'
url = f'{base}/{ticker}/history/'
x = requests.get(url).content
soup = bs4.BeautifulSoup(x, features='html.parser')
# https://stackoverflow.com/questions/39631386/how-to-understand-this-raw-html-of-yahoo-finance-when-retrieving-data-using-pyt
script = soup.find('script', text=re.compile('root.App.main')).text
j = json.loads(re.search('root.App.main\s+=\s+(\{.*\})', script).group(1),
parse_float=lambda x: x)
That’s it! The rest is just manipulating the json to get the fields of interest. Full source: [https://github.com/carlohamalainen/playground/blob/master/python/beautiful_soup_4/yahoo_stock_prices.py](https://github.com/carlohamalainen/playground/blob/master/python/beautiful_soup_4/yahoo_stock_prices.py
Sample run:
$ python yahoo_stock_prices.py
AUD to SGD: ('AUDSGD=X', 'Europe/London', '4:21PM BST', 0.9273)
BHP.AX AUD 2018-10-15 33.90
BHP.AX AUD 2018-10-16 33.66
BHP.AX AUD 2018-10-17 33.20
BHP.AX AUD 2018-10-18 33.10
BHP.AX AUD 2018-10-21 33.16
BHP.AX AUD 2018-10-22 32.79
BHP.AX AUD 2018-10-23 32.07
BHP.AX AUD 2018-10-24 30.80
BHP.AX AUD 2018-10-25 31.20